This week Matthias has let loose his signal processing tools to track the history of loudness in the UK singles charts. He shows in detail how pop music has become louder and flatter, and explains why loud doesn't have to be noisy.
There's no doubt that music keeps changing. The music psychologist Carol Krumhansl recently conducted an experiment in which she played 400 millisecond snippets of pop music to individual participants. Surprisingly, from this tiny amount of information her participants could often predict the decade the music had been created in - even if they did not recognise the track itself.
We assume that changes in musical style are motivated by fashion or social factors, and not due to developments in the recording or post-production process. For example, the rise of punk music (as traced in my second blog post) appears to have been triggered mostly by social factors. Old-school disco bloomed and faded quickly, like a fashionable jeans cut. But when it comes to the 80s things are less clear: we suspect that the introduction of new technology, specifically drum machines, substantially changed dance music styles, and find some evidence for it (third blog post).
So, are music fashions caused by people wanting to listen to some music more than to other? - They like it, buy it, and it gets into the charts? Well, probably, but only until record producers found out that they could make people listen to their music, simply by making it louder, so it stands out from the crowd. And that's exactly what they did, spurred by the advent of the CD in the 1980s. Just as trees evolve to grow higher so they can outgrow other trees in the race for sunlight, music grew louder and louder in the race for attention. At least that's what people have found examining many examples of popular music. There's an excellent Wikipedia article on this phenomenon dubbed the Loudness War. Is the phenomenon really as wide-spread as we think it is? Can we really find increased loudness in the charts, and can we track down loudness's evil brother dynamic range compression?
The Higher Level
An audio engineer's measure of loudness is decibels relative to full scale (dBFS), and it's really just a measure of audio level. Essentially, dBFS is the logarithm of the energy in an audio waveform, minus the value of the loudest sine wave that you can fit on the recording medium (the “full scale”). So a full scale sine wave will have 0 dBFS, which is very loud, and most other sounds will have negative values.
If a music track is well-engineered, then the loudest samples of the waveform are already nearly at maximum level, so you can't make it much louder without changing the shape of the wave form, i.e. without making it sound different. If you still want the track to sound louder, you have to shape the wave form so that more parts of the signal approach maximum level. The route many mastering engineers have therefore taken is to squeeze the waveform peaks down and use the resulting room to blow the whole signal up again - this is dynamic range compression, and it means that the signal gets louder on average.
Below we have plotted the average dBFS value for tracks from the UK singles charts from 1964 to 2009, as a cloud of very light grey dots (the highest 5% and lowest 5% are hidden for better visualisation of the rest). In order not to get fooled by more or less normalised tracks, we subtracted every track's maximum dBFS value. The red curve we plotted on top is a local regression curve, complete with the dashed simultaneous confidence band (at 99% confidence, see Further Reading, below). The tight confidence band shows us that the real underlying curve is unlikely to be far from the one we estimated.
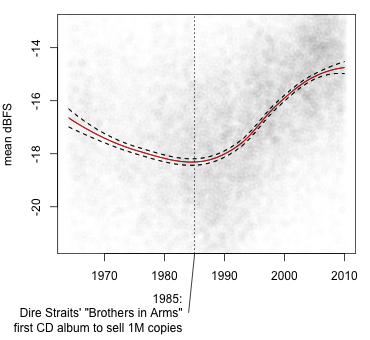
The average dBFS value for a typical 80s charts song was around -18 dBFS, but things have changed since then. Our data shows that the loudness war definitely happened, and it started shortly after the introduction of the CD in 1982. We marked Dire Straits' Brothers in Arms (1985), the first CD album that sold a million copies, in the plot. From there, it just goes up and up, to an average level of about -15 dBFS in 2009, or 3 dB higher than in the mid-80s. You can see that in the 70s, too, the dBFS values were relatively high. We believe that recordings of that time simply never had much dynamic range, due to limits of recording technology. Not everyone will agree with using dBFS as a measure for loudness though because it does not take into account the way humans perceive loudness at different frequencies. Do we have a measure for that, too?
It's Getting Louder all the Time
There are indeed computer algorithms that imitate how humans perceive loudness (see Further Reading). We applied such a measure of loudness to our singles charts tracks. Again, in order to make this measure independent from the maximum value used in the track we corrected for maximum dBFS value (this time by using linear prediction and plotting only the residuals). Measuring loudness this way shows a slightly different curve, an almost uninterrupted rise of loudness from the seventies through to the first decade of the 21st century (find the figure here). A more intuitive way of thinking about it is this: we take a loudness value that's very high at the beginning of our year range (in 1964), the 90% quantile. That means that only 10% of the charts in 1964 are louder than this value. We've then plotted the percentage of the tracks that were louder than the 1964 value for every year of the charts in the figure below.
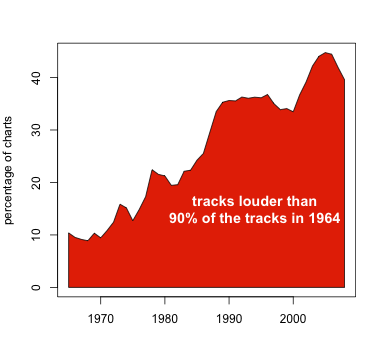
The percentage of loud tracks has increased from 10% in 1964 (by definition) to over 40% in recent years. So music has got louder. Well, isn't that in the spirit of Rock'n'Roll? Sadly, it isn't, because the increase in loudness has led to worse sound quality. Granted, it's louder, but boy is it flat!
The Death of Dynamic Range
If you fight the loudness war, whoever your competitors are, your victim will be dynamic range - an important part of sound quality. Here's why: I've already described to you the process of making a tracks sound louder by compressing the peaks, then blowing it up again. And the problem is just that: the peaks will sound compressed relative to the average recording. Drums have less punch, song sections intended to sound really loud will be at the same level as softer sections. This is all described very well in the Wikipedia article on the Loudness War. So we wanted to look at the development of dynamic range in the charts. We have actually measured the dynamic range of the music by a measure called crest. On every one-second block of a song, the crest is the difference in dB between the maximum value and the mean dBFS value. As in the figure above, we have plotted all tracks as a cloud of grey points, with the local regression and 99% confidence bands overlaid.
... and what we found exceeded our worst expectations. The charts tracks lost around 2 dB of dynamic range in the 20 years from 1985 to 2005 despite ever-improving technology, in energy terms that's 20% dynamic range lost. The picture is even more depressing when you look at individual artists. Madonna's tracks from the 80s have crest ranges of around 13.5 dB, with many tracks exceeding the average. But she goes with the loudness trend and gradually kills off 3dB of dynamic range, with her later recordings scoring around 10.5 dB. Hard to blame Madonna though, she's not alone! U2 seem to have resisted the trend for a long time, but then they fully embraced it, with their latest hits among the least dynamic of all. Oasis never had much dynamic range to begin with, so they've just kept on doing their thing, one might argue. Take That disbanded in 1996, then re-formed in 2005, but it looks as if they'd been going on all along: they're almost exactly on the loudness trend. Quite refreshingly, Robbie Williams and Moby do not seem to have followed the trend: while not topping the dynamic range chart, their tracks have actually grown more dynamic over time. And Beyoncé must be the queen of dynamics, most of her songs have well above the average dynamic range.
One of the arguments designed to convince artists not to make their music as loud as possible (beside the quality argument), is that modern software music players (including Last.fm's) adjust the volume so that loudness differences are less of an issue than they used to be. And some artists seem to have learned their lesson. The Red Hot Chili Peppers, for example, were criticised for their incredibly loud, and hence poor-in-dynamics, album Californication. Consider the very low dots with only approximately 9.5 dB dynamic range around the year 2000 in the figure above (with Red Hot Chili Peppers selected), way below even the average pop dynamic range. It's nice to see, however, that they steered back and their later recordings are more dynamic again.
compressed vs. dynamic
Red Hot Chili Peppers - Give It Away, 1991, very dynamic (15.0 dB crest)
Red Hot Chili Peppers - Californication, 2000, very compressed (9.2 dB crest)
Red Hot Chili Peppers - Dani California, 2006, quite dynamic again (11.2 dB crest)
“No, no, I mean, are they LOUD?”
You might have noticed that artists whose tracks have a low dynamic range are not necessarily renowned for being loud. Is Madonna louder these days than Beyoncé? The “loud” that we think of when talking about bands is concerned with the volumes involved while making the music, that is, however loud you mix a Take That track, Megadeth will sound louder. Cues for a band being really loud are distortion (distorted guitars in particular) and prominent cymbal sounds. The spectra of such sounds have the characteristic that spectral peaks are not very prominent, and that there's an emphasis on high frequency content. As it happens, we can measure these, too. Our inharmonicity metric measures the prominence of broadband noise relative to peaks in the spectrum, and high-frequency content is detected by a low 1st MFCC coefficient. Normalising these metrics and taking their geometric mean gives us a measure of noisiness. The picture below was compiled by positioning artist names by noisiness versus loudness. The font size and opacity of the names correspond to the number of singles in the UK charts.
So maybe the combination of loudness and noisiness gives you a better indication of the kind of “loud” that you like. Does it?
While today's post has been quite technical, next time we're going to look at the lighter side of things, with a special on songwriting.
Anatomy of the UK Charts series so far
Percussiveness and the Disco Diva - on the rise of disco in the mid 70s
Clash of Attitudes - on automatically telling punk from art rock
The Curse of the Drum Machine - on how 120 bpm dominated the 80s
Survival of the Flattest - this post
Further reading
The Wikipedia article on the loudness war.
The Echonest's Paul Lamere has also written a nice article showing evidence for the loudness war with many great examples.
Carol Krumhansl's article on the musical memory and the 400ms pop snippets: Plink: "Thin Slices" of Music, Carol L. Krumhansl, Music Perception: An Interdisciplinary Journal, Vol. 27, No. 5 (June 2010), pp. 337-354.
If you want to calculate loudness, inharmonicity and crest from a music file, try the libxtract Vamp plugin.
Local regression curves and confidences bands can be calculated using the locfit library, most easily using the interface to the R programming language.
Edit: The technical arm of the European Broadcasting Union EBU has a website with recommendations on the measurement and normalisation of loudness.